A simulation of Covid spread amongst all 5.7 million people in the South-West of England, enabled by the Hadean Distributed Cloud Platform
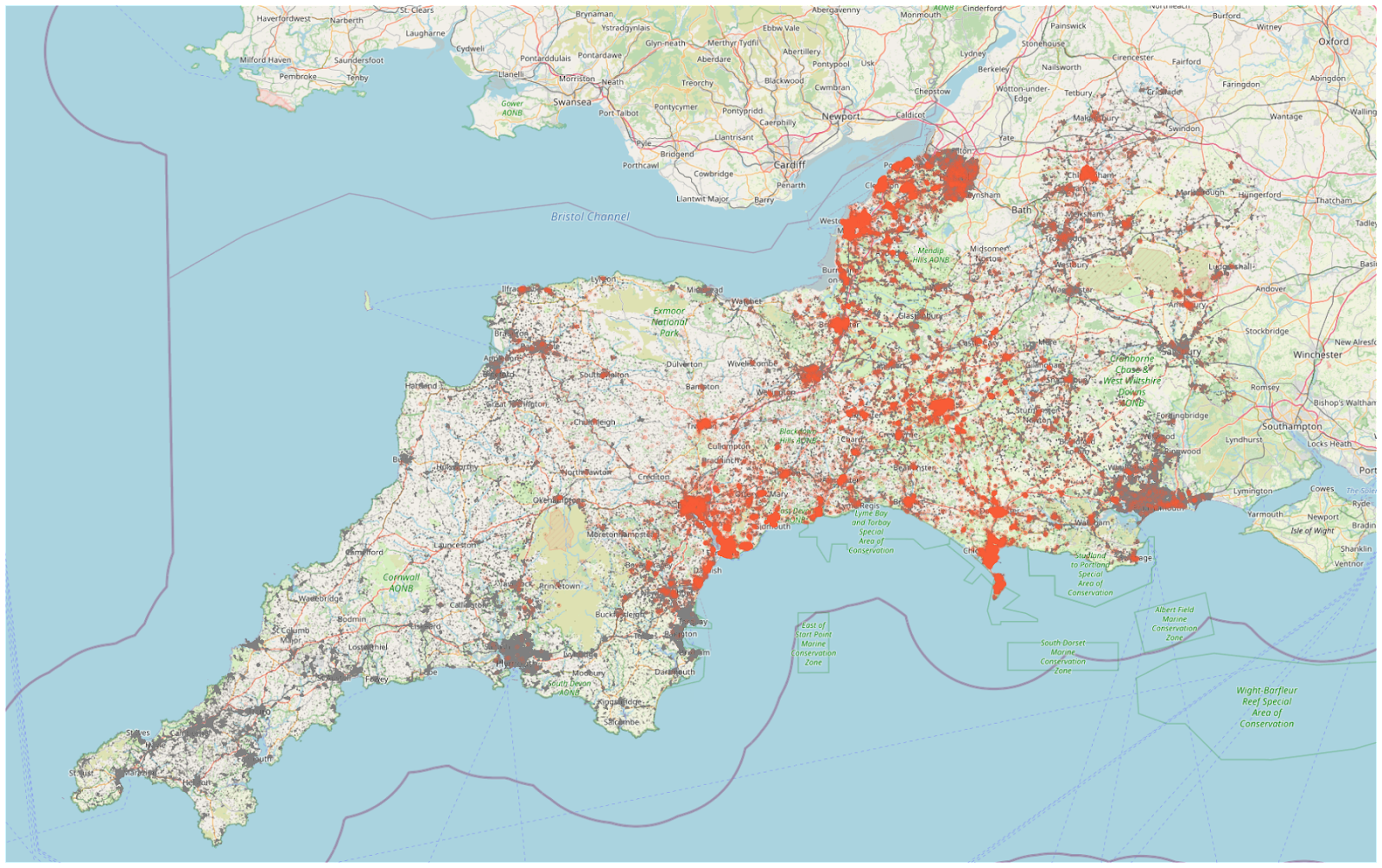
The turbulence caused by the pandemic has undoubtedly stressed the value of having a greater understanding of how different strategies and policies help us to respond to rare events. With each wave, we’ve benefited from our improved knowledge around slowing the spread – for example wearing masks or restricting travel. Despite this however, part of the struggle with Covid-19 is that more often than not, useful knowledge is often available too late. We desperately need solutions to observe all possible scenarios at a fast pace, such that we have time to react and provide optimal pandemic decision support.
Hadean’s Innovation team, in collaboration with epidemiologists at the University of Oxford and Imperial College London, have been creating models with this goal in mind. OpenABM is an open source agent-based simulation, which can use real world data to show the spread of Covid in a population. You can read about the technical details here. By default the solution is used to model the spread in the UK, though it can be re-parameterised for other countries. Using Hadean’s platform, the computation needed to run these simulations was scaled across many machines, achieving the fast rate of analysis needed for effective decision support.
But how exactly do these epidemiological models work? There are two main styles to mathematical epidemiology: compartmental models characterised by a small number of ordinary differential equations, and complex simulations of individual agents composing populations. Compartmental models are an important tool in giving high-level trends to infectious disease dynamics and can be fit to summary statistics of large populations to create inference of general trends. Although computationally simple to train, these models have difficulty in analysis of real-world policy. It is through multi-agent simulation that it becomes possible to accurately model, for example, social distancing, vaccine hesitancy, and geographical interventions. Though there is a step-change between the complexity to learn several characteristic equations, and to simulate millions of independent disease-spreading individuals. Further, creating insights by speculative simulation becomes intractable with the tools available to the average mathematical modeller.
Running these simulations has two key challenges: they’re intensive and need to be done quickly for the results to be useful. Distributed computing solutions generally have scaling issues due to bloated middleware and complex orchestration tools. Hadean’s distributed cloud platform, in contrast, removes this overhead and enables dynamic scaling of these high numbers of simulations. Thousands of virtual epidemics can be run in parallel, utilising as many machines as needed, using Hadean’s decision support system.
This method is highly beneficial for examining all the various what-if scenarios, particularly when wanting to examine a specific policy. Say for example you were particularly curious about looking at the value of performing “surge vaccinations” in a geographical radius about a particular outbreak or at-risk location. Previously, implementation of a new feature and the subsequent simulation and visualisation, would take significant amounts of time due to the computational complexity involved. Using the Hadean platform, this compute ‘heavy lifting’ is simplified to get results in hours.
In the image below we have a heatmap demonstrating this kind of analysis. We consider a representative network of a local authority in the UK, and seed an infection in a random household. The spatial intervention we introduce is a radial lockdown, that sends everyone in a radius, r (km), into quarantine after a delay, τ (days). To explore the efficacy of these lockdowns many independent simulations are needed to vary these parameters. Additionally, because the spread of covid is non-deterministic, Monte Carlo simulation is required for each parameter set. The summary statistic, the end result we are interested in is the total number of deaths. For a range of time delays and radii a heatmap is plotted. A dark colour indicates a large number of mortalities. From the detailed view afforded by Hadean’s decision support we can see, as expected, that the faster and stronger a lockdown is the more effective it is. Further it shows a stronger reliance on speed of intervention rather than strength. The Hadean platform allows not only a wider search of parameter space, but in repeating these individual runs also provides insight in the face of stochastic experiments. In this heatmap each cell represents the average of summary statistics of 4 independent runs of simulations which represent over 100k individuals spreading coronavirus for 64 days. For every extra day delay in implementation it can be seen that the lockdown radius needs to be increased by, of the order, 5km to totally suppress the new epidemic in this scenario.
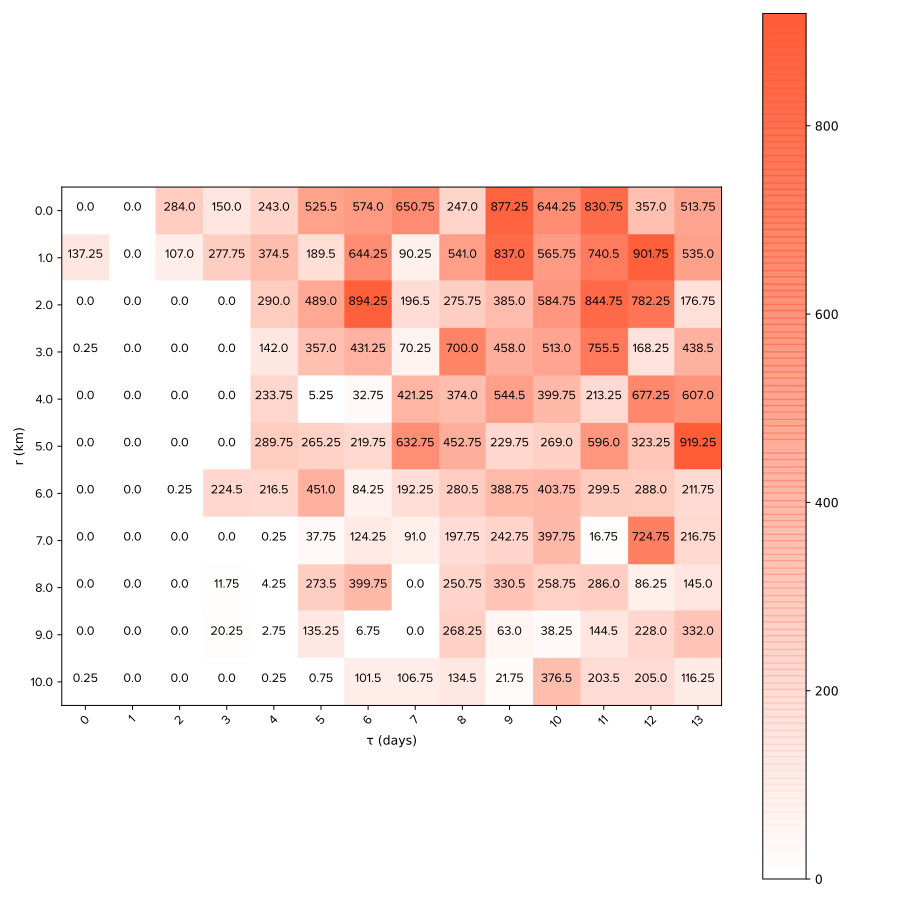
Furthermore, simulations of events in massive populations naturally can run into scaling issues simply due to the nature of the problem. In fact, when running a simulation of the spread in the south-west of England using a local laptop, the simulation crashes at around 100,000 individuals. In contrast, when running on the Hadean platform, 5.7 million people were modelled, with the results visualised using GIS, seen at the top of this page.
Beyond Pandemic Decision Support
The speed of analysis demonstrated here is helping drive other use cases for Hadean’s decision support system. After all, generating a swift response of course applies to a number of problems beyond the realm of epidemiology. For example, the events of the last couple years also showed the fragility of modern supply chains, where different approaches could lead to millions lost or gained. Foreseeing how every scenario plays out can help protect working capital, mitigate risk and ultimately enable the optimal fulfilment of KPIs. As we move forward, we’re excited to see how Hadean’s parallelised, live simulations can provide insight into a range of global problems beyond pandemic decision support.
A simulation of Covid spread amongst all 5.7 million people in the South-West of England, enabled by the Hadean Distributed Cloud Platform
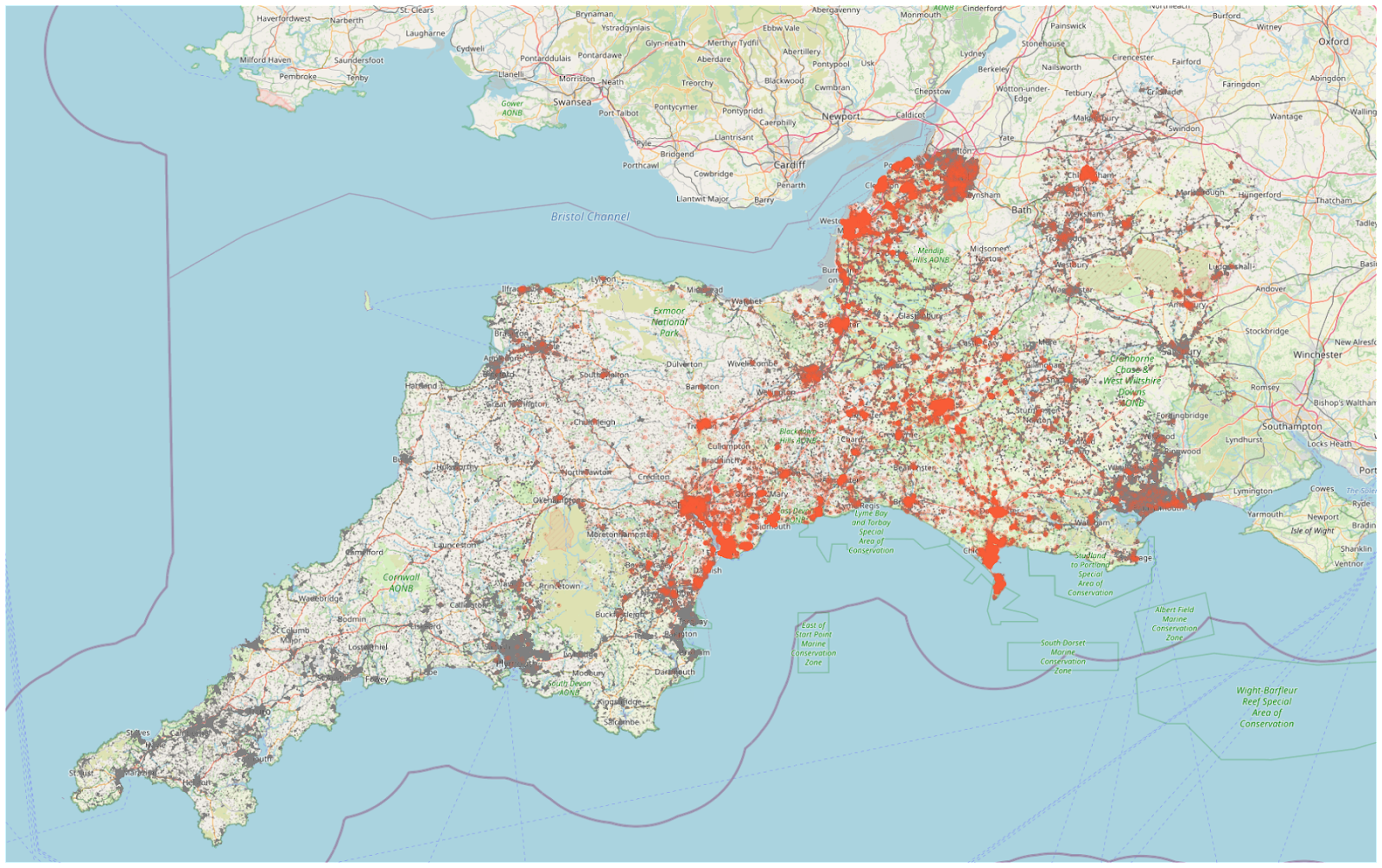
The turbulence caused by the pandemic has undoubtedly stressed the value of having a greater understanding of how different strategies and policies help us to respond to rare events. With each wave, we’ve benefited from our improved knowledge around slowing the spread – for example wearing masks or restricting travel. Despite this however, part of the struggle with Covid-19 is that more often than not, useful knowledge is often available too late. We desperately need solutions to observe all possible scenarios at a fast pace, such that we have time to react and provide optimal pandemic decision support.
Hadean’s Innovation team, in collaboration with epidemiologists at the University of Oxford and Imperial College London, have been creating models with this goal in mind. OpenABM is an open source agent-based simulation, which can use real world data to show the spread of Covid in a population. You can read about the technical details here. By default the solution is used to model the spread in the UK, though it can be re-parameterised for other countries. Using Hadean’s platform, the computation needed to run these simulations was scaled across many machines, achieving the fast rate of analysis needed for effective decision support.
But how exactly do these epidemiological models work? There are two main styles to mathematical epidemiology: compartmental models characterised by a small number of ordinary differential equations, and complex simulations of individual agents composing populations. Compartmental models are an important tool in giving high-level trends to infectious disease dynamics and can be fit to summary statistics of large populations to create inference of general trends. Although computationally simple to train, these models have difficulty in analysis of real-world policy. It is through multi-agent simulation that it becomes possible to accurately model, for example, social distancing, vaccine hesitancy, and geographical interventions. Though there is a step-change between the complexity to learn several characteristic equations, and to simulate millions of independent disease-spreading individuals. Further, creating insights by speculative simulation becomes intractable with the tools available to the average mathematical modeller.
Running these simulations has two key challenges: they’re intensive and need to be done quickly for the results to be useful. Distributed computing solutions generally have scaling issues due to bloated middleware and complex orchestration tools. Hadean’s distributed cloud platform, in contrast, removes this overhead and enables dynamic scaling of these high numbers of simulations. Thousands of virtual epidemics can be run in parallel, utilising as many machines as needed, using Hadean’s decision support system.
This method is highly beneficial for examining all the various what-if scenarios, particularly when wanting to examine a specific policy. Say for example you were particularly curious about looking at the value of performing “surge vaccinations” in a geographical radius about a particular outbreak or at-risk location. Previously, implementation of a new feature and the subsequent simulation and visualisation, would take significant amounts of time due to the computational complexity involved. Using the Hadean platform, this compute ‘heavy lifting’ is simplified to get results in hours.
In the image below we have a heatmap demonstrating this kind of analysis. We consider a representative network of a local authority in the UK, and seed an infection in a random household. The spatial intervention we introduce is a radial lockdown, that sends everyone in a radius, r (km), into quarantine after a delay, τ (days). To explore the efficacy of these lockdowns many independent simulations are needed to vary these parameters. Additionally, because the spread of covid is non-deterministic, Monte Carlo simulation is required for each parameter set. The summary statistic, the end result we are interested in is the total number of deaths. For a range of time delays and radii a heatmap is plotted. A dark colour indicates a large number of mortalities. From the detailed view afforded by Hadean’s decision support we can see, as expected, that the faster and stronger a lockdown is the more effective it is. Further it shows a stronger reliance on speed of intervention rather than strength. The Hadean platform allows not only a wider search of parameter space, but in repeating these individual runs also provides insight in the face of stochastic experiments. In this heatmap each cell represents the average of summary statistics of 4 independent runs of simulations which represent over 100k individuals spreading coronavirus for 64 days. For every extra day delay in implementation it can be seen that the lockdown radius needs to be increased by, of the order, 5km to totally suppress the new epidemic in this scenario.
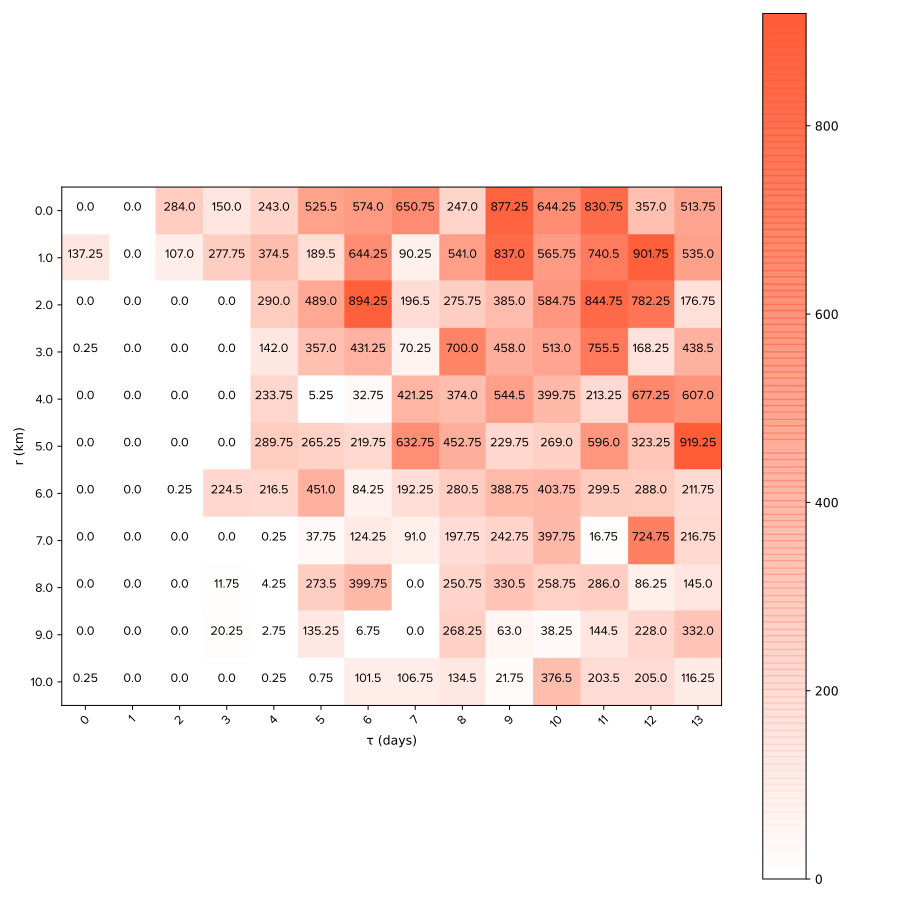
Furthermore, simulations of events in massive populations naturally can run into scaling issues simply due to the nature of the problem. In fact, when running a simulation of the spread in the south-west of England using a local laptop, the simulation crashes at around 100,000 individuals. In contrast, when running on the Hadean platform, 5.7 million people were modelled, with the results visualised using GIS, seen at the top of this page.
Beyond Pandemic Decision Support
The speed of analysis demonstrated here is helping drive other use cases for Hadean’s decision support system. After all, generating a swift response of course applies to a number of problems beyond the realm of epidemiology. For example, the events of the last couple years also showed the fragility of modern supply chains, where different approaches could lead to millions lost or gained. Foreseeing how every scenario plays out can help protect working capital, mitigate risk and ultimately enable the optimal fulfilment of KPIs. As we move forward, we’re excited to see how Hadean’s parallelised, live simulations can provide insight into a range of global problems beyond pandemic decision support.